The goal of this work is to recognize egocentric
atomic events in real-time. The atomic events are characterised
in terms of binary relationships (
bag-of-relations) between parts of the body and manipulated
objects. The key contribution is to summarise, within a histogram,
the relationships that hold over fixed time interval. This histogram is
then classfied into one of a number of atomic events. The relationships
encode both the types of body parts and objects involved (e.g. wrist,
hammer) together with a quantised representation of their distance apart
and the normalised rate of change in this distance. The quantisation and
classifier are both configured in a prior learning phase from training data.
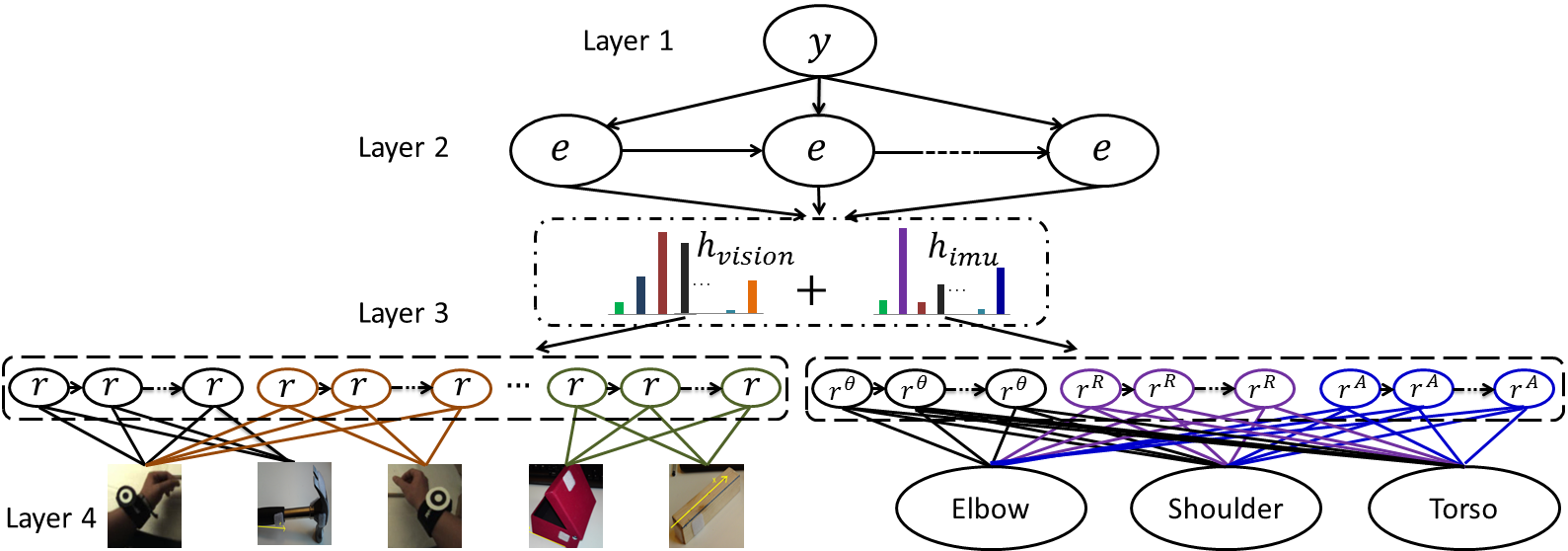
Overview of our hierarchical framework: atomic events
e are inferred using
spatiotemporal pairwise relations
r from observed objects and wrists, and relations between body parts (
elbow-shoulder
and
shoulder-torso) using inertia sensors (IMU). Activities
y are represented as a set of temporally-consistent
e.
Motivation
Most of the existing approaches for activity recognition are designed to
perform after-the-fact classification of activities after fully
observing videos of single activity. Moreover, such systems usually expect that the
same number of people or objects are observed over the entire activity whilst in
realistic scenarios often people and objects enter/leave the scene while activity is
going on.
There are three main objectives of the proposed activity recognition system: 1) to recognise the current event from a short
observation period (typically two seconds); 2) to anticipate the most probable
event that follows on from the current event; 3) to recognise activity deviations.
Publications
A. Behera, A. G. Cohn, D. C. Hogg. Workflow Activity Monitoring using the Dynamics of Pair-wise Qualitative
Spatial Relations. International Conference on MultiMedia Modeling (MMM 2012) (Oral).
pdf
Demos: driving screws and
hammering nails
S. F. Worgan, A. Behera, A. G. Cohn, D. C. Hogg. Exploiting petri-net structure for activity
classification and user instruction within an industrial setting.
International Conference on Multimodal Interaction (ICMI 2011).
pdf |
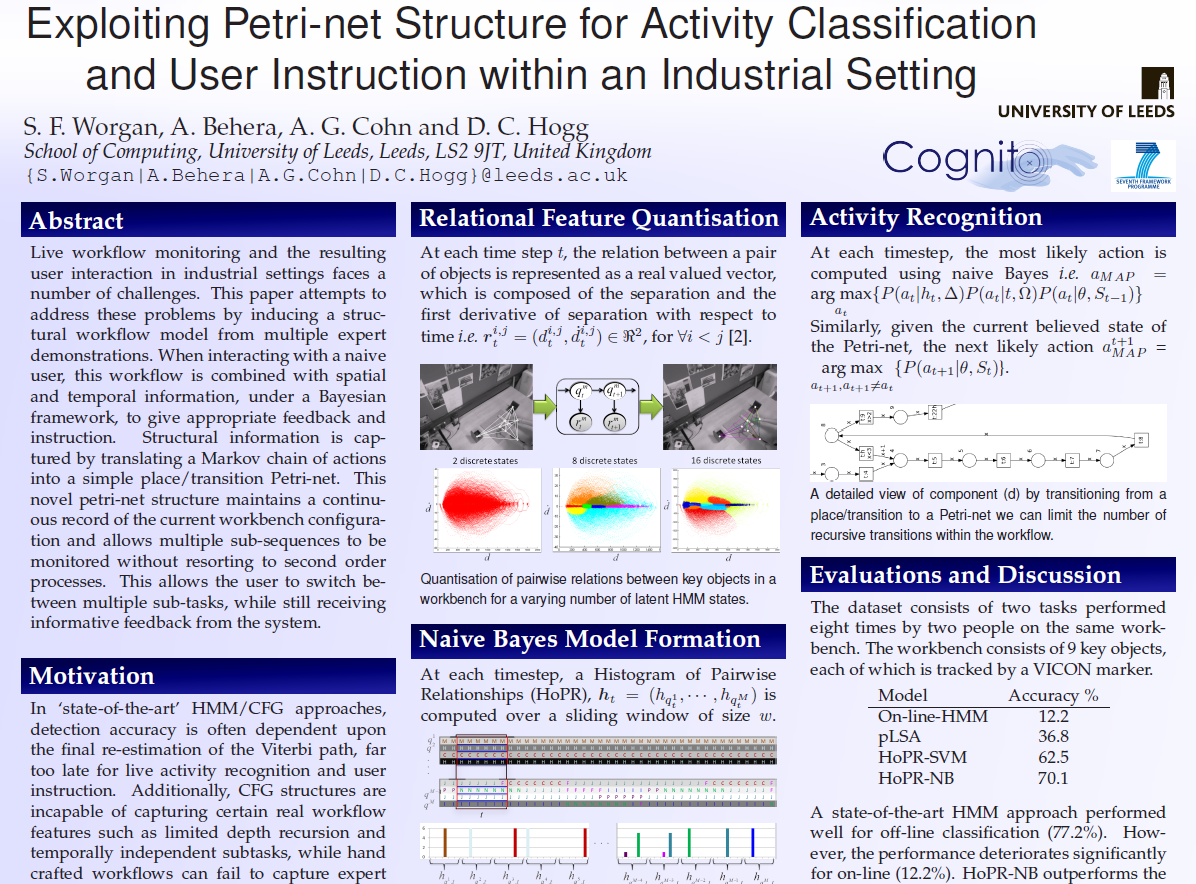 poster |
Datasets
In order to test our hierarchical framework, we have obtained two datasets using
an egocentric setup. These datasets consist of non-periodic manipulative tasks
in an industrial context. All the sequences were captured with on-body sensors
consisting IMUs, a backpack-mounted RGB-D camera for top-view and a chestmounted
fisheye camera for front-view of the workbench.
The first dataset is the scenario of hammering nails and driving screws.
In this dataset, subjects are asked to hammer 3 nails and drive 3 screws
using prescribed tools.
The second dataset is a labelling and packaging bottles scenario. In this
dataset, participants asked to attach labels to two bottles, then package them
in the correct positions within a box. This requires opening the box, placing
the bottles, closing the box, and then writing on the box as completed using a
marker pen.
Hammering three nails and driving three screws
Snapshots from the dataset. The first two images of are from
the top view (RGB-D) and the last two are from the chest-view fisheye camera.
The dataset consists of videos from both views, object tracklets (3D positions), upperbody model (IMU) and ground-truth.
The top view video sequences (~0.7 GB) and front
view sequences (~0.6 GB) are available.
Object and wrist tracklets, and IMU data (upperbody model) and activity labels are available here (~80MB).
Labelling and packaging bottles
Snapshots from the dataset. The first two images of are from
the top view (RGB-D) and the last two are from the chest-view fisheye camera.
The dataset consists of videos from both views, object tracklets (3D positions), upperbody model (IMU) and ground-truth.
The top view video sequences and the activity labels are available here (~1.7 GB).
Objects and wrist tracklets, and IMU data (upperbody model) can be found here (~100MB).
Acknowledgement
This research work is supported by EU FP7 (ICT Cognitive
Systems and Robotics) grant on
COGNITO (ICT-
248290) project. We also thank our collaborators at the
COGNITO partners.